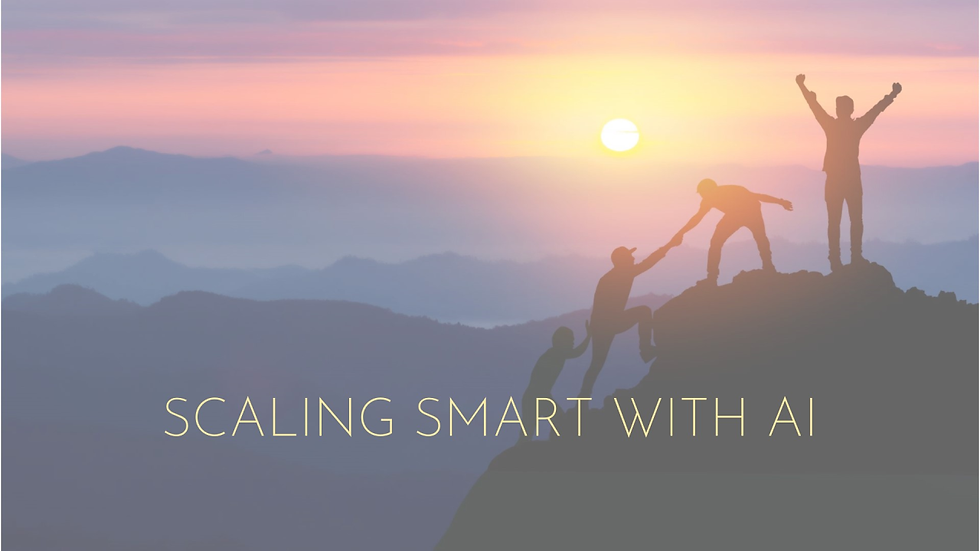
Step 1: Start Small and Deliver Value
Embarking on an AI inititative doesn’t mean tackling everything at once. The best way to begin is by focusing on delivering immediate, tangible value. We start with simple automations—like data entry or handling routine customer queries. These are low-risk wins that quickly improve efficiency and demonstrate the potential of AI, setting the stage for more complex projects.
Recent studies on AI adoption show that starting with core capabilities such as natural language understanding and data processing helps get quick, actionable feedback and eases the path into larger integrations.
Step 2: Expand to Bigger Opportunities
Once we prove the value with some early wins, it is time to aim higher. We identify manual, repetitive, high-volume tasks—perfect candidates for automation that offer substantial benefits. For example, deploying chat-to-agent models that can run specific tools or process data through natural language prompts. This is where scaling starts to take off. The key is to layer complexity gradually, using advanced methods like Retrieval-Augmented Generation (RAG) or Parameter Efficient Fine Tuning (PEFT), to ensure our system becomes more robust without overwhelming our team or infrastructure.
Step 3: Scale Smart with Iteration
Scaling effectively means being iterative and data-driven. We break projects into smaller, achievable steps, evaluate their impact, and refine our approach as we progress. For industries with sensitive data, we find that using synthetic datasets is a smart way to maintain privacy while enhancing AI capabilities. A modular approach allows us to manage risk and continuously improve both the technology and the team’s skill set. For mission-critical AI, incorporating human-in-the-loop checks helps boost trust and reliability in each iteration, paving the way for broader, more autonomous implementations.
Scaling AI is more of a steady climb than a giant leap. We start with small but meaningful wins, and let each success build momentum for the next. Automating straightforward tasks shows their value, and serves as the foundation for future growth. Before long, AI becomes an integral part of our business, driving efficiency and growth in ways that reshape our operations.
Comments